Business Intelligence and Analytics: Systems for Decision Support, Global Edition
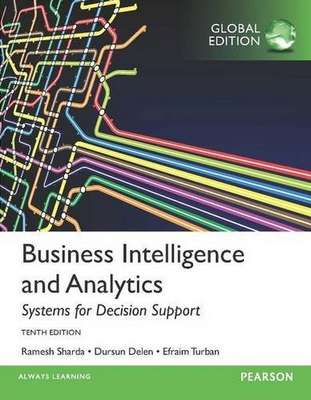
Námskeið
- V-874-REK5 Business Intelligence and Analytics
Lýsing:
Appropriate for all courses in Decision Support Systems (DSS), computerised decision making tools, and management support systems. Decision Support and Business Intelligence Systems provides the only comprehensive, up-to-date guide to today's revolutionary management support system technologies, and showcases how they can be used for better decision-making. The 10th edition focuses on Business Intelligence (BI) and analytics for enterprise decision support in a more streamlined book.
In addition to traditional decision support applications, this edition expands the reader’s understanding of the various types of analytics by providing examples, products, services, and exercises by discussing Web-related issues throughout the text. The full text downloaded to your computer With eBooks you can: search for key concepts, words and phrases make highlights and notes as you study share your notes with friends eBooks are downloaded to your computer and accessible either offline through the Bookshelf (available as a free download), available online and also via the iPad and Android apps.
Annað
- Höfundar: Efraim Turban, Ramesh Sharda, Dursun Delen
- Útgáfa:10
- Útgáfudagur: 2014-09-10
- Blaðsíður: 688
- Hægt að prenta út 2 bls.
- Hægt að afrita 2 bls.
- Format:Page Fidelity
- ISBN 13: 9781292009261
- Print ISBN: 9781292009209
- ISBN 10: 1292009268
Efnisyfirlit
- Title Page
- Contents
- Preface
- About the Authors
- Part I Decision Making and Analytics: An Overview
- Chapter 1 An Overview of Business Intelligence, Analytics, and Decision Support
- 1.1 Opening Vignette: Magpie Sensing Employs Analytics to Manage a Vaccine Supply Chain Effectively
- 1.2 Changing Business Environments and Computerized Decision Support
- The Business Pressures–Responses–Support Model
- 1.3 Managerial Decision Making
- The Nature of Managers’ Work
- The Decision-Making Process
- 1.4 Information Systems Support for Decision Making
- 1.5 An Early Framework for Computerized Decision Support
- The Gorry and Scott-Morton Classical Framework
- Computer Support for Structured Decisions
- Computer Support for Unstructured Decisions
- Computer Support for Semistructured Problems
- 1.6 The Concept of Decision Support Systems (DSS)
- DSS as an Umbrella Term
- Evolution of DSS into Business Intelligence
- 1.7 A Framework for Business Intelligence (BI)
- Definitions of BI
- A Brief History of BI
- The Architecture of BI
- Styles of BI
- The Origins and Drivers of BI
- A Multimedia Exercise in Business Intelligence
- Application Case 1.1 Sabre Helps Its Clients Through Dashboards and Analytics
- The DSS–BI Connection
- 1.8 Business Analytics Overview
- Descriptive Analytics
- Application Case 1.2 Eliminating Inefficiencies at Seattle Children’s Hospital
- Application Case 1.3 Analysis at the Speed of Thought
- Predictive Analytics
- Application Case 1.4 Moneyball: Analytics in Sports and Movies
- Application Case 1.5 Analyzing Athletic Injuries
- Prescriptive Analytics
- Application Case 1.6 Industrial and Commercial Bank of China (ICBC) Employs Models to Reconfigure It
- Analytics Applied to Different Domains
- Analytics or Data Science?
- 1.9 Brief Introduction to Big Data Analytics
- What Is Big Data?
- Application Case 1.7 Gilt Groupe’s Flash Sales Streamlined by Big Data Analytics
- 1.10 Plan of the Book
- Part I: Business Analytics: An Overview
- Part II: Descriptive Analytics
- Part III: Predictive Analytics
- Part IV: Prescriptive Analytics
- Part V: Big Data and Future Directions for Business Analytics
- 1.11 Resources, Links, and the Teradata University Network Connection
- Resources and Links
- Vendors, Products, and Demos
- Periodicals
- The Teradata University Network Connection
- The Book’s Web Site
- Chapter Highlights
- Key Terms
- Questions for Discussion
- Exercises
- End-of-Chapter Application Case Nationwide Insurance Used BI to Enhance Customer Service
- References
- Chapter 1 An Overview of Business Intelligence, Analytics, and Decision Support
- Chapter 2 Foundations and Technologies for Decision Making
- 2.1 Opening Vignette: Decision Modeling at HP Using Spreadsheets
- 2.2 Decision Making: Introduction and Definitions
- Characteristics of Decision Making
- A Working Definition of Decision Making
- Decision-Making Disciplines
- Decision Style and Decision Makers
- 2.3 Phases of the Decision-Making Process
- 2.4 Decision Making: The Intelligence Phase
- Problem (or Opportunity) Identification
- Application Case 2.1 Making Elevators Go Faster!
- Problem Classification
- Problem Decomposition
- Problem Ownership
- 2.5 Decision Making: The Design Phase
- Models
- Mathematical (Quantitative) Models
- The Benefits of Models
- Selection of a Principle of Choice
- Normative Models
- Suboptimization
- Descriptive Models
- Good Enough, or Satisficing
- Developing (Generating) Alternatives
- Measuring Outcomes
- Risk
- Scenarios
- Possible Scenarios
- Errors in Decision Making
- 2.6 Decision Making: The Choice Phase
- 2.7 Decision Making: The Implementation Phase
- 2.8 How Decisions Are Supported
- Support for the Intelligence Phase
- Support for the Design Phase
- Support for the Choice Phase
- Support for the Implementation Phase
- 2.9 Decision Support Systems: Capabilities
- A DSS Application
- 2.10 DSS Classifications
- The AIS SIGDSS Classification for DSS
- Other DSS Categories
- Custom-Made Systems Versus Ready-Made Systems
- 2.11 Components of Decision Support Systems
- The Data Management Subsystem
- The Model Management Subsystem
- Application Case 2.2 Station Casinos Wins by Building Customer Relationships Using Its Data
- Application Case 2.3 SNAP DSS Helps OneNet MakeTelecommunications Rate Decisions
- The User Interface Subsystem
- The Knowledge-Based Management Subsystem
- Application Case 2.4 From a Game Winner to a Doctor!
- Chapter Highlights
- Key Terms
- Questions for Discussion
- Exercises
- End-of-Chapter Application Case Logistics Optimization in a Major Shipping Company (CSAV)
- References
- Chapter 3 Data Warehousing
- 3.1 Opening Vignette: Isle of Capri Casinos Is Winning with Enterprise Data Warehouse
- 3.2 Data Warehousing Definitions and Concepts
- What Is a Data Warehouse?
- A Historical Perspective to Data Warehousing
- Characteristics of Data Warehousing
- Data Marts
- Operational Data Stores
- Enterprise Data Warehouses (EDW)
- Metadata
- Application Case 3.1 A Better Data Plan: Well-Established TELCOs Leverage Data Warehousing and Analy
- 3.3 Data Warehousing Process Overview
- Application Case 3.2 Data Warehousing Helps MultiCare Save More Lives
- 3.4 Data Warehousing Architectures
- Alternative Data Warehousing Architectures
- Which Architecture Is the Best?
- 3.5 Data Integration and the Extraction, Transformation, and Load (ETL) Processes
- Data Integration
- Application Case 3.3 BP Lubricants Achieves BIGS Success
- Extraction, Transformation, and Load
- 3.6 Data Warehouse Development
- Application Case 3.4 Things Go Better with Coke’s Data Warehouse
- Data Warehouse Development Approaches
- Application Case 3.5 Starwood Hotels & Resorts Manages Hotel Profitability with Data Warehousing
- Additional Data Warehouse Development Considerations
- Representation of Data in Data Warehouse
- Analysis of Data in the Data Warehouse
- OLAP Versus OLTP
- OLAP Operations
- 3.7 Data Warehousing Implementation Issues
- Application Case 3.6 EDW Helps Connect State Agencies in Michigan
- Massive Data Warehouses and Scalability
- 3.8 Real-Time Data Warehousing
- Application Case 3.7 Egg Plc Fries the Competition in Near Real Time
- 3.9 Data Warehouse Administration, Security Issues, and Future Trends
- The Future of Data Warehousing
- 3.10 Resources, Links, and the Teradata University Network Connection
- Resources and Links
- Cases
- Vendors, Products, and Demos
- Periodicals
- Additional References
- The Teradata University Network (TUN) Connection
- Chapter Highlights
- Key Terms
- Questions for Discussion
- Exercises
- End-of-Chapter Application Case Continental Airlines Flies High with Its Real-Time Data Warehouse
- References
- 4.1 Opening Vignette:Self-Service Reporting Environment Saves Millions for Corporate Customers
- 4.2 Business Reporting Definitions and Concepts
- What Is a Business Report?
- Application Case 4.1 Delta Lloyd Group Ensures Accuracy and Efficiency in Financial Reporting
- Components of the Business Reporting System
- Application Case 4.2 Flood of Paper Ends at FEMA
- 4.3 Data and Information Visualization
- Application Case 4.3 Tableau Saves Blastrac Thousands of Dollars with Simplified Information Sharing
- A Brief History of Data Visualization
- Application Case 4.4 TIBCO Spotfire Provides Dana-Farber Cancer Institute with Unprecedented Insight
- 4.4 Different Types of Charts and Graphs
- Basic Charts and Graphs
- Specialized Charts and Graphs
- 4.5 The Emergence of Data Visualization and Visual Analytics
- Visual Analytics
- High-Powered Visual Analytics Environments
- 4.6 Performance Dashboards
- Application Case 4.5 Dallas Cowboys Score Big with Tableau and Teknion
- Dashboard Design
- Application Case 4.6 Saudi Telecom Company Excels with Information Visualization
- What to Look For in a Dashboard
- Best Practices in Dashboard Design
- Benchmark Key Performance Indicators with Industry Standards
- Wrap the Dashboard Metrics with Contextual Metadata
- Validate the Dashboard Design by a Usability Specialist
- Prioritize and Rank Alerts/Exceptions Streamed to the Dashboard
- Enrich Dashboard with Business Users’ Comments
- Present Information in Three Different Levels
- Pick the Right Visual Construct Using Dashboard Design Principles
- Provide for Guided Analytics
- 4.7 Business Performance Management
- Closed-Loop BPM Cycle
- Application Case 4.7 IBM Cognos Express Helps Mace for Faster
- 4.8 Performance Measurement
- Key Performance Indicator (KPI)
- Performance Measurement System
- 4.9 Balanced Scorecards
- The Four Perspectives
- The Meaning of Balance in BSC
- Dashboards Versus Scorecards
- 4.10 Six Sigma as a Performance Measurement System
- The DMAIC Performance Model
- Balanced Scorecard Versus Six Sigma
- Effective Performance Measurement
- Application Case 4.8 Expedia.com’s Customer Satisfaction Scorecard
- Chapter Highlights
- Key Terms
- Questions for Discussion
- Exercises
- End-of-Chapter Application Case Smart Business Reporting Helps Healthcare Providers Deliver Better C
- References
- Chapter 5 Data Mining
- 5.1 Opening Vignette: Cabela’s Reels in More Customers withAdvanced Analytics and Data Mining
- 5.2 Data Mining Concepts and Applications
- Application Case 5.1 Smarter Insurance: Infinity P&C ImprovesCustomer Service and Combats Fraud with
- Definitions, Characteristics, and Benefits
- Application Case 5.2 Harnessing Analytics to Combat Crime:Predictive Analytics Helps Memphis Police
- How Data Mining Works
- Data Mining Versus Statistics
- 5.3 Data Mining Applications
- Application Case 5.3 A Mine on Terrorist Funding
- 5.4 Data Mining Process
- Step 1: Business Understanding
- Step 2: Data Understanding
- Step 3: Data Preparation
- Step 4: Model Building
- Application Case 5.4 Data Mining in Cancer Research
- Step 5: Testing and Evaluation
- Step 6: Deployment
- Other Data Mining Standardized Processes and Methodologies
- 5.5 Data Mining Methods
- Classification
- Estimating the True Accuracy of Classification Models
- Cluster Analysis for Data Mining
- Application Case 5.5 2degrees Gets a 1275 Percent Boost in ChurnIdentification
- Association Rule Mining
- 5.6 Data Mining Software Tools
- Application Case 5.6 Data Mining Goes to Hollywood: PredictingFinancial Success of Movies
- 5.7 Data Mining Privacy Issues, Myths, and Blunders
- Data Mining and Privacy Issues
- Application Case 5.7 Predicting Customer Buying Patterns—TheTarget Story
- Data Mining Myths and Blunders
- Chapter Highlights
- Key Terms
- Questions for Discussion
- Exercises
- End-of-Chapter Application Case Macys.com Enhances ItsCustomers’ Shopping Experience with Analytic
- References
- 6.1 Opening Vignette: Predictive Modeling Helps BetterUnderstand and Manage Complex MedicalProcedure
- 6.2 Basic Concepts of Neural Networks
- Biological and Artificial Neural Networks
- Application Case 6.1 Neural Networks Are Helping to Save Lives inthe Mining Industry
- Elements of ANN
- Network Information Processing
- Neural Network Architectures
- Application Case 6.2 Predictive Modeling Is Powering the PowerGenerators
- 6.3 Developing Neural Network–Based Systems
- The General ANN Learning Process
- Backpropagation
- 6.4 Illuminating the Black Box of ANN with SensitivityAnalysis
- Application Case 6.3 Sensitivity Analysis Reveals Injury SeverityFactors in Traffic Accidents
- 6.5 Support Vector Machines
- Application Case 6.4 Managing Student Retention with PredictiveModeling
- Mathematical Formulation of SVMs
- Primal Form
- Dual Form
- Soft Margin
- Nonlinear Classification
- Kernel Trick
- 6.6 A Process-Based Approach to the Use of SVM
- Support Vector Machines Versus Artificial Neural Networks
- 6.7 Nearest Neighbor Method for Prediction
- Similarity Measure: The Distance Metric
- Parameter Selection
- Application Case 6.5 Efficient Image Recognition andCategorization with kNN
- Chapter Highlights
- Key Terms
- Questions for Discussion
- Exercises
- End-of-Chapter Application Case Coors Improves Beer Flavorswith Neural Networks
- References
- 7.1 Opening Vignette: Machine Versus Men on Jeopardy!: TheStory of Watson
- 7.2 Text Analytics and Text Mining Concepts andDefinitions
- Application Case 7.1 Text Mining for Patent Analysis
- 7.3 Natural Language Processing
- Application Case 7.2 Text Mining Improves Hong KongGovernment’s Ability to Anticipate and Address
- 7.4 Text Mining Applications
- Marketing Applications
- Security Applications
- Application Case 7.3 Mining for Lies
- Biomedical Applications
- Academic Applications
- Application Case 7.4 Text Mining and Sentiment Analysis HelpImprove Customer Service Performance
- 7.5 Text Mining Process
- Task 1: Establish the Corpus
- Task 2: Create the Term–Document Matrix
- Task 3: Extract the Knowledge
- Application Case 7.5 Research Literature Survey with TextMining
- 7.6 Text Mining Tools
- Commercial Software Tools
- Free Software Tools
- Application Case 7.6 A Potpourri of Text Mining Case Synopses
- 7.7 Sentiment Analysis Overview
- Application Case 7.7 Whirlpool Achieves Customer Loyalty andProduct Success with Text Analytics
- 7.8 Sentiment Analysis Applications
- 7.9 Sentiment Analysis Process
- Methods for Polarity Identification
- Using a Lexicon
- Using a Collection of Training Documents
- Identifying Semantic Orientation of Sentences and Phrases
- Identifying Semantic Orientation of Document
- 7.10 Sentiment Analysis and Speech Analytics 359How Is It Done?
- Application Case 7.8 Cutting Through the Confusion: Blue CrossBlue Shield of North Carolina Uses Nex
- Chapter Highlights
- Key Terms
- Questions for Discussion
- Exercises
- End-of-Chapter Application Case BBVA Seamlessly Monitorsand Improves Its Online Reputation
- References
- 8.1 Opening Vignette: Security First Insurance Deepens Connection with Policyholders
- 8.2 Web Mining Overview
- 8.3 Web Content and Web Structure Mining
- Application Case 8.1 Identifying Extremist Groups with Web Linkand Content Analysis
- 8.4 Search Engines
- Anatomy of a Search Engine
- 1. Development Cycle
- Web Crawler
- Document Indexer
- 2. Response Cycle
- Query Analyzer
- Document Matcher/Ranker
- How Does Google Do It?
- Application Case 8.2 IGN Increases Search Traffic by 1500 Percent
- 8.5 Search Engine Optimization
- Methods for Search Engine Optimization
- Application Case 8.3 Understanding Why Customers Abandon Shopping Carts Results in $10 Million Sales
- 8.6 Web Usage Mining (Web Analytics)
- Web Analytics Technologies
- Application Case 8.4 Allegro Boosts Online Click-Through Rates by 500 Percent with Web Analysis
- Web Analytics Metrics
- Web Site Usability
- Traffic Sources
- Visitor Profiles
- Conversion Statistics
- 8.7 Web Analytics Maturity Model and Web Analytics Tools
- Web Analytics Tools
- Putting It All Together—A Web Site Optimization Ecosystem
- A Framework for Voice of the Customer Strategy
- 8.8 Social Analytics and Social Network Analysis
- Social Network Analysis
- Social Network Analysis Metrics
- Application Case 8.5 Social Network Analysis HelpsTelecommunication Firms
- Connections
- Distributions
- Segmentation
- 8.9 Social Media Definitions and Concepts
- How Do People Use Social Media?
- Application Case 8.6 Measuring the Impact of Social Media at Lollapalooza
- 8.10 Social Media Analytics
- Measuring the Social Media Impact
- Best Practices in Social Media Analytics
- Application Case 8.7 eHarmony Uses Social Media to Help Take the Mystery Out of Online Dating
- Social Media Analytics Tools and Vendors
- Chapter Highlights
- Key Terms
- Questions for Discussion
- Exercises
- End-of-Chapter Application Case Keeping Students on Track with Web and Predictive Analytics
- References
- Chapter 9 Model-Based Decision Making: Optimization and Multi-Criteria Systems
- 9.1 Opening Vignette: Midwest ISO Saves Billions by Better Planning of Power Plant Operations and Ca
- 9.2 Decision Support Systems Modeling
- Application Case 9.1 Optimal Transport for ExxonMobil Downstream Through a DSS
- Current Modeling Issues
- Application Case 9.2 Forecasting/Predictive Analytics Proves to Bea Good Gamble for Harrah’s Chero
- 9.3 Structure of Mathematical Models for Decision Support
- The Components of Decision Support Mathematical Models
- The Structure of Mathematical Models
- 9.4 Certainty, Uncertainty, and Risk
- Decision Making Under Certainty
- Decision Making Under Uncertainty
- Decision Making Under Risk (Risk Analysis)
- Application Case 9.3 American Airlines UsesShould-Cost Modeling to Assess the Uncertainty of Bidsfor
- 9.5 Decision Modeling with Spreadsheets
- Application Case 9.4 Showcase Scheduling at Fred Astaire East Side Dance Studio
- 9.6 Mathematical Programming Optimization
- Application Case 9.5 Spreadsheet Model Helps Assign Medical Residents
- Mathematical Programming
- Linear Programming
- Modeling in LP: An Example
- Implementation
- 9.7 Multiple Goals, Sensitivity Analysis, What-If Analysis,and Goal Seeking
- Multiple Goals
- Sensitivity Analysis
- What-If Analysis
- Goal Seeking
- 9.8 Decision Analysis with Decision Tables and Decision Trees
- Decision Tables
- Decision Trees
- 9.9 Multi-Criteria Decision Making With Pairwise Comparisons
- The Analytic Hierarchy Process
- Application Case 9.6 U.S. HUD Saves the House by Using AHP for Selecting IT Projects
- Tutorial on Applying Analytic Hierarchy Process Using Web-HIPRE
- Chapter Highlights
- Key Terms
- Questions for Discussion
- Exercises
- End-of-Chapter Application Case Pre-Positioning of Emergency Items for CARE International
- References
- 10.1 Opening Vignette: System Dynamics Allows FluorCorporation to Better Plan for Project and Change
- 10.2 Problem-Solving Search Methods
- Analytical Techniques
- Algorithms
- Blind Searching
- Heuristic Searching
- Application Case 10.1 Chilean Government Uses Heuristics to Make Decisions on School Lunch Providers
- 10.3 Genetic Algorithms and Developing GA Applications
- Example: The Vector Game
- Terminology of Genetic Algorithms
- How Do Genetic Algorithms Work?
- Limitations of Genetic Algorithms
- Genetic Algorithm Applications
- 10.4 Simulation
- Application Case 10.2 Improving Maintenance Decision Making in the Finnish Air Force Through Simulat
- Application Case 10.3 Simulating Effects of Hepatitis B Interventions
- Major Characteristics of Simulation
- Advantages of Simulation
- Disadvantages of Simulation
- The Methodology of Simulation
- Simulation Types
- Monte Carlo Simulation
- Discrete Event Simulation
- 10.5 Visual Interactive Simulation
- Conventional Simulation Inadequacies
- Visual Interactive Simulation
- Visual Interactive Models and DSS
- Application Case 10.4 Improving Job-Shop Scheduling DecisionsThrough RFID: A Simulation-Based Assess
- Simulation Software
- 10.6 System Dynamics Modeling
- 10.7 Agent-Based Modeling
- Application Case 10.5 Agent-Based Simulation Helps Analyze Spread of a Pandemic Outbreak
- Chapter Highlights
- Key Terms
- Questions for Discussion
- Exercises
- End-of-Chapter Application Case HP Applies Management Science Modeling to Optimize Its Supply Chain
- References
- 11.1 Opening Vignette: InterContinental Hotel Group Uses Decision Rules for Optimal Hotel Room Rates
- 11.2 Automated Decision Systems
- Application Case 11.1 Giant Food Stores Prices the EntireStore
- 11.3 The Artificial Intelligence Field
- 11.4 Basic Concepts of Expert Systems
- Experts
- Expertise
- Features of ES
- Application Case 11.2 Expert System Helps in Identifying SportTalents
- 11.5 Applications of Expert Systems
- Application Case 11.3 Expert System Aids in Identification of Chemical, Biological, and Radiological
- Classical Applications of ES
- Newer Applications of ES
- Areas for ES Applications
- 11.6 Structure of Expert Systems
- Knowledge Acquisition Subsystem
- Knowledge Base
- Inference Engine
- User Interface
- Blackboard (Workplace)
- Explanation Subsystem (Justifier)
- Knowledge-Refining System
- Application Case 11.4 Diagnosing Heart Diseases by Signal Processing
- 11.7 Knowledge Engineering
- Knowledge Acquisition
- Knowledge Verification and Validation
- Knowledge Representation
- Inferencing
- Explanation and Justification
- 11.8 Problem Areas Suitable for Expert Systems
- 11.9 Development of Expert Systems
- Defining the Nature and Scope of the Problem
- Identifying Proper Experts
- Acquiring Knowledge
- Selecting the Building Tools
- Coding the System
- Evaluating the System
- Application Case 11.5 Clinical Decision Support System for Tendon Injuries
- 11.10 Concluding Remarks
- Chapter Highlights
- Key Terms
- Questions for Discussion
- Exercises
- End-of-Chapter Application Case Tax Collections Optimization for New York State
- References
- 12.1 Opening Vignette: Expertise Transfer System to Train Future Army Personnel
- 12.2 Introduction to Knowledge Management
- Knowledge Management Concepts and Definitions
- Knowledge
- Explicit and Tacit Knowledge
- 12.3 Approaches to Knowledge Management
- The Process Approach to Knowledge Management
- The Practice Approach to Knowledge Management
- Hybrid Approaches to Knowledge Management
- Knowledge Repositories
- 12.4 Information Technology (IT) in Knowledge Management
- The KMS Cycle
- Components of KMS
- Technologies That Support Knowledge Management
- 12.5 Making Decisions in Groups: Characteristics, Process,Benefits, and Dysfunctions
- Characteristics of Groupwork
- The Group Decision-Making Process
- The Benefits and Limitations of Groupwork
- 12.6 Supporting Groupwork with Computerized Systems
- An Overview of Group Support Systems (GSS)
- Groupware
- Time/Place Framework
- 12.7 Tools for Indirect Support of Decision Making
- Groupware Tools
- Groupware
- Collaborative Workflow
- Web 2.0
- Wikis
- Collaborative Networks
- 12.8 Direct Computerized Support for Decision Making:From Group Decision Support Systems to Group Su
- Group Decision Support Systems (GDSS)
- Group Support Systems
- How GDSS (or GSS) Improve Groupwork
- Facilities for GDSS
- Chapter Highlights
- Key Terms
- Questions for Discussion
- Exercises
- End-of-Chapter Application Case Solving Crimes by Sharing Digital Forensic Knowledge
- References
- Chapter 13 Big Data and Analytics
- 13.1 Opening Vignette: Big Data Meets Big Science at CERN
- 13.2 Definition of Big Data
- The Vs That Define Big Data
- Application Case 13.1 Big Data Analytics Helps Luxottica ImproveIts Marketing Effectiveness
- 13.3 Fundamentals of Big Data Analytics
- Business Problems Addressed by Big Data Analytics
- Application Case 13.2 Top 5 Investment Bank Achieves Single Source of Truth
- 13.4 Big Data Technologies
- MapReduce
- Why Use MapReduce?
- Hadoop
- How Does Hadoop Work?
- Hadoop Technical Components
- Hadoop: The Pros and Cons
- NoSQL
- Application Case 13.3 eBay’s Big Data Solution
- 13.5 Data Scientist
- Where Do Data Scientists Come From?
- Application Case 13.4 Big Data and Analytics in Politics
- 13.6 Big Data and Data Warehousing
- Use Case(s) for Hadoop
- Use Case(s) for Data Warehousing
- The Gray Areas (Any One of the Two Would Do the Job)
- Coexistence of Hadoop and Data Warehouse
- 13.7 Big Data Vendors
- Application Case 13.5 Dublin City Council Is Leveraging Big Datato Reduce Traffic Congestion
- Application Case 13.6 Creditreform Boosts Credit Rating Quality with Big Data Visual Analytics
- 13.8 Big Data and Stream Analytics
- Stream Analytics Versus Perpetual Analytics
- Critical Event Processing
- Data Stream Mining
- 13.9 Applications of Stream Analytics
- e-Commerce
- Telecommunications
- Application Case 13.7 Turning Machine-Generated Streaming Data into Valuable Business Insights
- Law Enforcement and Cyber Security
- Power Industry
- Financial Services
- Health Sciences
- Government
- Chapter Highlights
- Key Terms
- Questions for Discussion
- Exercises
- End-of-Chapter Application Case Discovery Health Turns Big Data into Better Healthcare
- References
- 14.1 Opening Vignette: Oklahoma Gas and Electric Employs Analytics to Promote Smart Energy Use
- 14.2 Location-Based Analytics for Organizations
- Geospatial Analytics
- Application Case 14.1 Great Clips Employs Spatial Analytics to Shave Time in Location Decisions
- A Multimedia Exercise in Analytics Employing Geospatial Analytics
- Real-Time Location Intelligence
- Application Case 14.2 Quiznos Targets Customers for Its Sandwiches
- 14.3 Analytics Applications for Consumers
- Application Case 14.3 A Life Coach in Your Pocket
- 14.4 Recommendation Engines
- 14.5 Web 2.0 and Online Social Networking
- Representative Characteristics of Web 2.0
- Social Networking
- A Definition and Basic Information
- Implications of Business and Enterprise Social Networks
- 14.6 Cloud Computing and BI
- Service-Oriented DSS
- Data-as-a-Service (DaaS)
- Information-as-a-Service (Information on Demand) (IaaS)
- Analytics-as-a-Service (AaaS)
- 14.7 Impacts of Analytics in Organizations: An Overview
- New Organizational Units
- Restructuring Business Processes and Virtual Teams
- The Impacts of ADS Systems
- Job Satisfaction
- Job Stress and Anxiety
- Analytics’ Impact on Managers’ Activities and Their Performance
- 14.8 Issues of Legality, Privacy, and Ethics
- Legal Issues
- Privacy
- Recent Technology Issues in Privacy and Analytics
- Ethics in Decision Making and Support
- 14.9 An Overview of the Analytics Ecosystem
- Analytics Industry Clusters
- Data Infrastructure Providers
- Data Warehouse Industry
- Middleware Industry
- Data Aggregators/Distributors
- Analytics-Focused Software Developers
- Reporting/Analytics
- Predictive Analytics
- Prescriptive Analytics
- Application Developers or System Integrators: Industry Specific or General
- Analytics User Organizations
- Analytics Industry Analysts and Influencers
- Academic Providers and Certification Agencies
- Chapter Highlights
- Key Terms
- Questions for Discussion
- Exercises
- End-of-Chapter Application Case Southern States Cooperative Optimizes Its Catalog Campaign
- References
- A
- B
- C
- D
- E
- F
- G
- H
- I
- J
- K
- L
- M
- N
- O
- P
- Q
- R
- S
- T
- U
- V
- W
- X
- Y
UM RAFBÆKUR Á HEIMKAUP.IS
Bókahillan þín er þitt svæði og þar eru bækurnar þínar geymdar. Þú kemst í bókahilluna þína hvar og hvenær sem er í tölvu eða snjalltæki. Einfalt og þægilegt!Rafbók til eignar
Rafbók til eignar þarf að hlaða niður á þau tæki sem þú vilt nota innan eins árs frá því bókin er keypt.
Þú kemst í bækurnar hvar sem er
Þú getur nálgast allar raf(skóla)bækurnar þínar á einu augabragði, hvar og hvenær sem er í bókahillunni þinni. Engin taska, enginn kyndill og ekkert vesen (hvað þá yfirvigt).
Auðvelt að fletta og leita
Þú getur flakkað milli síðna og kafla eins og þér hentar best og farið beint í ákveðna kafla úr efnisyfirlitinu. Í leitinni finnur þú orð, kafla eða síður í einum smelli.
Glósur og yfirstrikanir
Þú getur auðkennt textabrot með mismunandi litum og skrifað glósur að vild í rafbókina. Þú getur jafnvel séð glósur og yfirstrikanir hjá bekkjarsystkinum og kennara ef þeir leyfa það. Allt á einum stað.
Hvað viltu sjá? / Þú ræður hvernig síðan lítur út
Þú lagar síðuna að þínum þörfum. Stækkaðu eða minnkaðu myndir og texta með multi-level zoom til að sjá síðuna eins og þér hentar best í þínu námi.
Fleiri góðir kostir
- Þú getur prentað síður úr bókinni (innan þeirra marka sem útgefandinn setur)
- Möguleiki á tengingu við annað stafrænt og gagnvirkt efni, svo sem myndbönd eða spurningar úr efninu
- Auðvelt að afrita og líma efni/texta fyrir t.d. heimaverkefni eða ritgerðir
- Styður tækni sem hjálpar nemendum með sjón- eða heyrnarskerðingu
- Gerð : 208
- Höfundur : 6180
- Útgáfuár : 2014
- Leyfi : 380